Home / The Essential Guide to AI Management
The Essential Guide to AI Management
AI management is the process of establishing policies, procedures, and standardized protocols for the responsible use of AI deployment within an organization. In recent years, we’ve seen AI integration as a truly transformative force across many industries, helping organizations to enhance efficiency, make data-driven decisions, and improve customer and employee experiences. However, with the rapid integration of AI enablement technologies comes the need for effective AI management practices. This article delves into the importance of properly managing an AI model, featured components, challenges it addresses, and best practices.
What is AI Management?
AI management follows a structured approach to overseeing AI connectivity and technologies within an organization. It involves setting guidelines that ensure ethical, transparent, and accountable use of AI orchestration across systems while simultaneously addressing risks and opportunities associated with these technologies. The goal is to maintain and continuously improve the AI Enablement Platform over time, ensuring it adapts to changing business needs and societal expectations.
Key Components of AI Management
- AI Management Systems (AIMS): These frameworks help organizations manage the risks and opportunities associated with AI deployment. They include guidelines for ethics, accountability, transparency, and data privacy. By implementing an AIMS, organizations can ensure that their AI connectivity initiatives align with best practices and regulatory requirements.
- AI Data Management: This aspect focuses on using an enterprise AI platform and machine learning (ML) to automate and streamline data-related processes such as access, preparation, and secure exposure of enterprise knowledge. Effective data management is critical for training accurate AI models and making informed decisions based on reliable data.
- AI Field Management: This involves utilizing AI orchestration to efficiently manage inventory and assets. Organizations can automate tasks such as creating purchase orders and assigning them to warehouses through intelligent inventory management systems.
Why is AI Management Important?
The significance of effective AI management cannot be overstated. Here are three reasons why it plays a crucial role in modern organizations:
- Responsible and Sustainable Deployment: AI management helps ensure that AI deployment is done responsibly and sustainably. Organizations can mitigate the potential negative impacts of AI enablement technologies by establishing clear ethical policies and accountability practices.
- Efficiency and Cost-Effectiveness: Robust AI management practices allow organizations to streamline operations, reduce costs, and improve overall efficiency. By effectively managing AI resources while maximizing ROI, businesses can adapt quickly to changing market demands.
- Addressing Challenges Related to AI: AI integration comes with inherent challenges, such as privacy concerns, algorithm bias, and inequalities in outcomes. Effective management frameworks help organizations navigate these complexities by providing guidelines for ethical use, risk assessment, and continuous improvement.
Deep Dive into the Components of Effective AI Management
To implement successful AI management practices within an organization, several components should be considered.
Establishing an AIMS
An AIMS provides a structured framework for overseeing all aspects of AI deployment within an organization. As enterprises increasingly integrate AI technology into their operations, effective AIMS are critical. AIMS helps manage the complexities associated with AI technologies and ensures they align with ethical standards and organizational goals. This section explores key elements of an AIMS, including ethical principles, governance structures, and risk management processes, and discusses their importance in fostering responsible AI practices.
Ethical Principles
Ethical principles form the foundation of any AIMS. These guidelines ensure that enterprise AI platforms and algorithms are developed and used in a manner that respects human rights and societal values. The importance of ethics in AI cannot be overstated; as AI enablement platforms become more prevalent, the potential for misuse, bias, or unintended consequences increases. Ethical principles navigate these challenges by providing a framework for responsible decision-making.
Embedding these ethical principles into the AIMS, fosters a culture of responsibility and integrity surrounding AI integration initiatives, ultimately enhancing public trust and compliance as AI adoption increases.
- Fairness: Fairness aims to prevent algorithmic bias in an enterprise AI platform and ensure equitable treatment across diverse populations. Organizations must actively work to identify and mitigate biases in their datasets and algorithms to avoid perpetuating existing inequalities.
- Transparency: Transparency is crucial for building trust among stakeholders at all levels. Organizations should strive to make their enterprise AI platform understandable to users by documenting the algorithms’ decision-making processes and making this information accessible to relevant parties.
- Accountability: Establishing accountability is essential for ensuring that individuals or teams are credited for the outcomes produced by the enterprise AI platform. Organizations should define clear roles and responsibilities related to AI deployment and development, allowing for effective oversight and recourse in case of adverse outcomes.
- Privacy: Protecting user privacy is a fundamental ethical consideration in AI deployment. Organizations must implement robust data protection measures to safeguard sensitive information from unauthorized access or misuse, adhering to relevant regulations such as GDPR or CCPA.
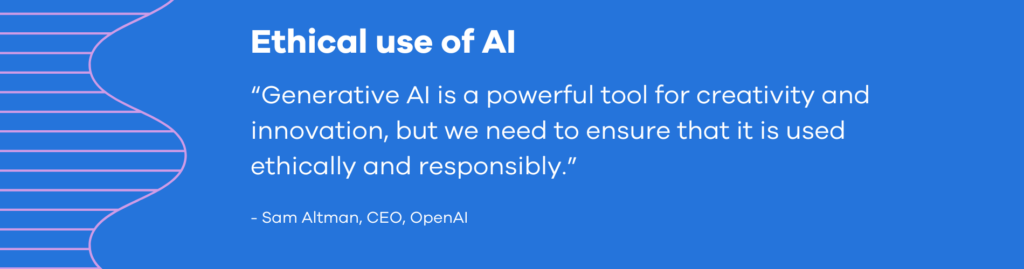
Governance Structures
Governance structures are critical components of an AIMS. They providing mechanisms for overseeing AI enablement platforms and maintaining compliance with ethical standards and legal requirements. Effective governance helps organizations manage the complexities associated with AI deployment while promoting accountability and transparency.
Organizations can effectively oversee their AI connectivity activities by implementing robust governance structures within their AIMS while ensuring alignment with ethical principles and regulatory requirements.
- Leadership Roles: Establishing clear leadership roles within the governance structure is essential for effective oversight. Enterprises should appoint individuals or teams responsible for managing AI integration initiatives, such as a Chief AI Officer or an AI Governance Board. These leaders should deeply understand the technical aspects of AI enablement and organizational goals.
- Cross-Functional Collaboration: An effective governance structure encourages collaboration among various departments, including IT, legal, compliance, and business units. Organizations can develop a holistic approach to managing AI risks and opportunities by involving stakeholders from different areas of expertise.
- Policies and Procedures: Governance structures should include well-defined policies and procedures that guide AI orchestration, development, monitoring, and analysis. This may involve establishing protocols for regular audits, performance evaluations, and compliance checks to ensure adherence to ethical standards.
- Stakeholder Engagement: Engaging stakeholders throughout the governance process fosters transparency and accountability. Organizations should establish channels for communication between leadership, employees, users, and external parties to gather feedback on AI deployment initiatives and address concerns promptly.
Risk Management Processes
Risk management processes are integral to an AIMS, providing procedures for identifying, assessing, and mitigating risks associated with AI systems. As organizations adopt more advanced technologies, understanding potential risks becomes increasingly important to safeguard against adverse outcomes.
By integrating comprehensive risk management processes into their AIMS framework, organizations can proactively address potential challenges associated with AI management while safeguarding their interests and those of their stakeholders.
- Risk Identification: The first step in risk management involves identifying potential risks related to AI deployment. This includes evaluating technical risks (e.g., algorithmic failures), operational risks (e.g., integration challenges), legal risks (e.g., non-compliance with regulations), and reputational risks (e.g., public backlash).
- Risk Assessment: Once risks have been identified, enterprises must assess their potential impact on operations and stakeholders, by analyzing the probability of each risk occurring and the likely consequences if it does materialize.
- Mitigation Strategies: After assessing risks, organizations should develop mitigation strategies tailored to address specific vulnerabilities identified during the assessment phase. This may involve implementing technical safeguards (e.g., redundancy measures), establishing clear policies (e.g., usage guidelines), or conducting regular training sessions for employees on best practices related to AI integration.
- Continuous Monitoring: Risk management is not a single effort; it requires ongoing analysis of both internal systems and external factors that may impact AI connectivity initiatives over time. Organizations should establish mechanisms for tracking performance metrics related to risk management efforts while remaining vigilant about emerging threats or changes in regulatory landscapes.
Implementing Robust Data Management Practices
Data is the cornerstone of an effective AI enablement platform; hence managing it properly is vital. The quality, accessibility, and security of data directly influence the performance of AI models and the insights they generate. To ensure that organizations can leverage their data effectively, robust data management practices must be implemented. This section will explore key strategies grouped into three phases: Access, Prepare, and Expose.
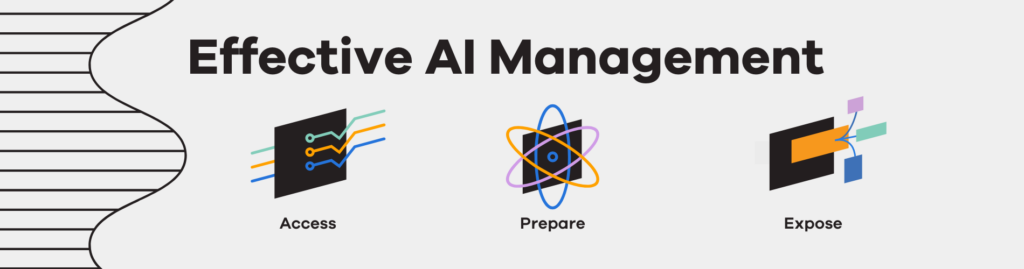
Phase 1: Access
The Access phase encompasses data collection and integration processes, which are crucial for establishing a solid foundation to manage data. Managing access to data lays the groundwork for high-quality datasets, which are essential for successful AI integration.
- Data Collection: Automating the collection process from various sources can significantly reduce the time and effort required for manual entry. Organizations can use APIs and automated scripts to gather data from internal systems, third-party applications, and external databases. This accelerates the data collection process and eliminates human errors that result from manually inputting data.
- Data Integration: Integrating data from disparate sources into a centralized repository allows enterprises to break down silos and gain a holistic view of their operations. Automated AI integration tools can streamline this process by continuously syncing real-time data across systems. This ensures that stakeholders have access to up-to-date information, facilitating informed decision-making.
Phase 2: Prepare
The Prepare phase focuses on data cleaning and transformation, ensuring it’s accurate, consistent, and ready for analysis. By prioritizing the Prepare phase, organizations ensure that their datasets are clean and structured to maximize their analytical potential.
- Data Cleaning: Data cleaning is essential for maintaining high-quality datasets. Automated tools can identify and rectify inaccuracies, such as duplicate entries, missing values, or inconsistent formatting. By employing ML algorithms, organizations can continuously monitor and improve the data quality over time. This ensures only accurate and relevant information is used in AI orchestration, leading to more reliable predictions.
- Data Transformation: Once cleaned, data often requires transformation to be suitable for analysis. Automated processes can standardize formats, aggregate information from multiple sources, and enrich datasets with additional attributes or metadata. This step is crucial for preparing data for ML algorithms, as it helps create a unified view that enhances analytical capabilities.
Phase 3: Expose
The Expose phase involves data analysis and visualization, allowing organizations to derive insights from their prepared datasets. By focusing on the Expose phase, organizations can effectively communicate insights from their data while empowering stakeholders to make informed decisions based on actionable insights.
- Data Analysis: Advanced analytics tools powered by AI can automate the analysis of large datasets, identifying trends, correlations, and patterns that may not be immediately apparent by manual examination. By leveraging ML algorithms for predictive analytics, organizations can forecast future outcomes based on historical data.
- Data Visualization: Automated visualization tools enable organizations to present complex data in an easily digestible format. Dashboards and interactive reports give stakeholders insights at a glance, allowing them to make quick decisions based on real-time information.
Leveraging Field Management Techniques
An enterprise AI platform enhances inventory and asset management by automating processes, streamlining operations, improving accuracy, and optimizing resource utilization. Two key techniques in this domain are demand forecasting and automated replenishment, which help enterprises maintain optimal inventory levels, contributing to overall operational efficiency.
- Demand Forecasting: Demand forecasting is a critical inventory management component. By utilizing predictive analytics, businesses can anticipate future inventory needs based on historical trends and current market conditions. This proactive approach allows organizations to optimize stock levels and reduce the risk of overstock or understock situations.
- Historical Data Analysis: AI-driven demand forecasting begins by analyzing historical sales data. Examining patterns over time helps organizations identify seasonal trends, customer behaviors, and other factors that influence demand. For example, a retail business may analyze sales data from previous holiday seasons to predict future demand.
- Incorporating External Factors: Beyond historical data, AI systems can incorporate external variables such as economic indicators, weather forecasts, and social media trends into their predictive AI models. This multifaceted approach enhances the accuracy of estimates by considering a broader range of influences on consumer behavior. For instance, during a heatwave, an ice cream manufacturer might anticipate a surge and adjust their inventory accordingly.
- Continuous Learning: One of the most significant advantages of AI in demand forecasting is its ability to learn continuously from new data. As market conditions change or new trends emerge, AI algorithms can quickly adapt their predictions. This dynamic capability ensures that businesses remain agile and responsive to fluctuations in demand, ultimately leading to better decision making.
- Optimizing Inventory Levels: By predicting future demand, companies can determine optimal inventory levels to meet customer needs without incurring excess carrying costs. This balance minimizes capital tied up in unsold goods while ensuring products are available when customers want them.
Automated Replenishment
Automated replenishment is another powerful technique that leverages AI enablement to streamline inventory management processes. Intelligent systems can automatically reorder stock when levels fall below predefined thresholds, ensuring businesses maintain optimal inventory levels without manual intervention.
- Integration with Supply Chain Management: Automated replenishment systems can be integrated with broader supply chain management solutions to enhance overall operational efficiency. By synchronizing inventory levels with supplier capabilities and logistics operations, businesses can create a more responsive supply chain that adapts quickly to changing demands.
- Real-Time Monitoring: AI-powered systems continuously monitor inventory levels in real time. By integrating with point-of-sale systems and warehouse management software, these systems can track stock movements and sales patterns as they occur. This real-time visibility allows for timely replenishment actions based on current inventory status.
- Dynamic Reorder Points: Traditional replenishment methods rely on static reorder points based on historical averages. However, AI systems adjust these reorder points dynamically based on predictive analytics and current market conditions. For example, if a product experiences an unexpected spike in sales due to a marketing campaign, the enterprise AI platform can automatically lower the reorder point to prevent stockouts.
- Streamlining Purchase Orders: An automated replenishment system triggers reorder requests and generates purchase orders for suppliers based on predefined criteria such as lead times and supplier performance metrics. This automation reduces the administrative burden on staff and eliminates human error in the ordering process.
- Cost Efficiency: By optimizing the timing and quantity of orders through automated replenishment, organizations can reduce holding costs associated with excess inventory while ensuring they have enough stock to meet customer demand promptly. This efficiency translates into improved cash flow and profitability.
Challenges in AI Management
While the benefits of effective AI management are clear, three challenges must be addressed.
1. The Ethical Use of AI
As organizations deploy more advanced technologies like ML algorithms or natural language processing (NLP) tools, they must remain vigilant about ethical considerations:
- Bias Mitigation: Organizations should actively work to identify biases in their data sets or algorithms that could lead to unfair outcomes.
- Transparency: Giving stakeholders insight into how the AI enablement platform makes decisions fosters trust among users.
2. Navigating Regulatory Compliance
With regulations surrounding data privacy (such as HIPAA) and ethical considerations in technology deployment:
- Staying Informed: Organizations must keep abreast of changing laws affecting their use of artificial intelligence.
- Implementing Compliance Frameworks: Establishing compliance protocols ensures adherence to legal requirements while minimizing risks associated with non-compliance.
3. Managing Change Effectively
Integrating new technologies often requires significant organizational change:
- Change Management Strategies: Implementing change management frameworks can help ease transitions by preparing employees for new processes or tools.
- Training Programs: Providing training sessions ensures staff members are equipped with the skills to utilize new systems effectively.
Best Practices for Successful AI Management
To maximize success when deploying AI connectivity, consider these best practices:
- Involve Stakeholders Early: Engaging stakeholders throughout the development process helps align expectations and ensures that deployed solutions effectively meet user needs.
- Focus on Explainability: Developing interpretable models fosters trust among users, and providing explanations for predictions helps stakeholders understand how the enterprise AI search platform makes decisions.
- Establish Clear Metrics for Success: Define success metrics up front so everyone involved understands what constitutes successful deployment. This could include user adoption rates or improvements in operational efficiency post-deployment.
- Implement Robust Testing Protocols: Rigorous testing before full-scale launch minimizes risks associated with unexpected behavior once deployed and includes stress-testing under scenarios simulated through test environments mimicking production settings closely enough without impacting live operations negatively during trials run beforehand, if possible.
- Create Feedback Mechanisms Post-deployment: Establish channels through which users can provide feedback about their experiences using deployed solutions; this feedback loop enables continuous improvement efforts over time while fostering engagement among end-users who feel heard.
AI management is essential for AI integration into modern organizations responsibly and sustainably. establishing robust policies around ethics, accountability, and transparency and implementing effective data management practices allows businesses to harness the full potential of their AI investment while rising to address common challenges and avoid pitfalls.